What is artificial intelligence?
Artificial intelligence (AI) refers to the simulation of human intelligence in machines that are programmed to think and learn like humans. AI aims to mimic human cognitive functions by understanding large amounts of data to improve clinical decision-making (Jiang et al., 2017). We are not new to the concept of AI and often perceive it as something ubiquitous that will inevitably find its place in healthcare practice. But what do we really know about AI? And how much do we not know about its current integration and use in the clinical nursing work that we do? Let us cast our lens and take a deep dive into what AI actually does and how it assists with our clinical practice and in the healthcare system.
Historical development of AI in health care
The historical development of AI in health care has been marked by significant milestones and advancements, reflecting the growth of AI technology and its integration into clinical practice. Below is an overview of its evolution:
1950s
Initial concepts
The concept of using computers to simulate intelligent behaviour and critical thinking was first described by Alan Turing in 1950 in the book Computers and Intelligence (Kaul, Enslin & Gross, 2020).
Six years later, John McCarthy described the term artificial intelligence (AI) as ‘the science and engineering of making intelligent machines’ (Kaul, Enslin & Gross, 2020).
1950s – 1970s
The early AI
The early AI was focused on developing machines that had the ability to make inferences or decisions that previously only humans could make. The first industrial robot arm (named Unimate) was able to follow step-by-step commands to handle heavy objects and perform repetitive tasks (Kaul, Enslin & Gross, 2020).
In 1966, Shakey, ‘the first electronic person,’ was developed as the first mobile robot to be able to interpret instructions. This was an important milestone in robotics and the future of AI (Kaul, Enslin & Gross, 2020).
1970s – 2000s
The AI winter
Most of this time period is referred to as the ‘AI Winter’ due to the period of reduced funding and interest in AI and, subsequently, fewer significant developments (Kaul, Enslin & Gross, 2020).
During the 1980s, Expert Systems (ES) were developed to assist in medical decision-making by mimicking the knowledge and decision-making skills of human experts. In the same period, AI applications expanded into medical imaging, like the early versions of Computer-Aided Diagnosis (CAD), which were employed to help radiologists detect abnormalities in X-rays and MRIs (Salem et al., 2021).
2000s – 2020s
Advancements in AI
The emergence of machine learning (ML) was pioneered during the early 2000s which enhanced the ability of AI systems to analyse large datasets. This period saw the integration of AI into various healthcare applications, including predictive analytics and personalised medicines (Rajpurkar et al., 2022).
Electronic Health Records (EHRs) emerged as the widespread health records management system, providing a rich source of data for AI, enabling more accurate predictions and efficient healthcare delivery.
Deep Learning, Personalised Medicine, and Clinical Decision Support (CDS) were developed during the early 2010s, significantly improving AI’s capabilities in processing and interpreting complex medical data, developing personalised treatment plans, and assisting healthcare providers in making clinical decisions (Askin et al., 2023; Ludervold & Ludervold, 2018; Sloane & Silva, 2019).
2020s – present
Generative AI and modern AI Applications
The explosion of Generative AI (GenAI) in the early 2020s ushered in a new era of tangible applications of AI in consumers and the healthcare system. GenAI, such as ChatGPT and DALL-E 2, started creating entirely new things, from realistic images to complex text formats.
The potential application of GenAI in medicine and health care currently focuses on drug discovery, personalised medicine and report generation, and for nursing, patient education, documentation, simulation and training, and predictive analytics (Zhang & Kamel Boulos, 2023).
Digital health and applications of AI in nursing practice
Digital health encompasses a wide range of technologies, including electronic health records (EHRs), telehealth and virtual care, wearable devices, and mobile health apps. In Australia, the adoption of these technologies has been accelerated by the initiatives led by the National Digital Health Strategy, which aims to create a seamless and secure digital health ecosystem (ADHA, 2016).
For nurses, this means more efficient management of patient data, improved communication with other healthcare professionals, and better monitoring of patients’ health statuses. This seamless access to comprehensive patient information enables quicker decision-making and enhances the accuracy of diagnoses and treatment plans. It can also facilitate coordinated care, which can reduce the risk of clinical errors and ensure that patients receive timely and appropriate interventions.
AI, as we know, is making significant inroads into the nursing profession. AI-driven tools and apps are constantly being developed to assist in various aspects of patient care and administrative tasks. We may not realise it, but AI tools have been around us for some time supporting the daily tasks of nurses to detect patterns, predict patient outcomes and enhance the quality of care. Here are some examples of the integration of AI in clinical settings:
- Clinical Decision Support System (CDSS)
An AI-powered tool that provides nurses with evidence-based recommendations and alerts, helping them identify potential health issues early and make accurate clinical decisions.
Example: Pop-up alerts when opening BTF observation charts in EMR when abnormal results have not been actioned (HealthShare, 2013). - Predictive analytics
Uses historical data, statistical algorithms, and machine learning (ML) to identify the likelihood of future health outcomes based on past data. The goal of predictive analytics is to go beyond understanding what has happened to provide the best assessment of what will happen in the future.
Example: Ainsoff Deterioration Index (ADI) predicts the likelihood of unexpected and sudden deterioration in the patient’s condition. Currently used in Central Adelaide Local Health District (CALHD) and Sydney Adventist Hospital (Hospital and Healthcare, 2023). - Telehealth and remote patient monitoring
COVID-19 has accelerated the adoption of telehealth in Australia. AI plays a vital role in telehealth platforms, facilitating remote patient monitoring and virtual consultation. This technology allows nurses to provide care to patients in remote areas, ensuring continuity of care.
Example: eHealth NSW’s Virtual Care – Remote Patient Monitoring Program (VC-RPM) focuses on patients with chronic conditions such as DM II, heart failure, and COPD. It uses AI to monitor patients’ vital signs and symptoms through connected devices (smart tablets, IR thermometers, etc.) to generate real-time data (eHealth NSW, 2023).
Generative AI in nursing
Generative AI (GenAI) is a type of artificial intelligence technology that can produce new content such as text, images, audio, and video based on the data it has been trained on. In 2022, OpenAI released the famous ‘ChatGPT’ – a data-driven revolutionary GenAI tool that summarises data into text for expedited information-gathering that is faster than popular Google internet search engines and content databases. Following the introduction of ChatGPT, other companies released similar solutions, such as Microsoft Bing (before Microsoft acquired OpenAI) and Google’s BardAI (now called Gemini) to enable users to access information at their fingertips. AI technology like this, once intangible for individuals, is now accessible in multiple industries, including health care (Caroll, 2023).
As nurses, how does GenAI impact our daily nursing tasks and patient care outcomes? There are some potential and future benefits and applications of GenAI for nurses:
- Enhancing clinical decision support
The rapid data access of GenAI can analyse clinical data quickly, providing nurses with handy, timely insights and recommendations about clinical decision-making in patient care. However, it is important to understand that the answers provided by GenAI are not always accurate, and it is important to verify information with credible sources. - Improved documentation and administrative efficiency
GenAI can transcribe spoken notes into written documents, significantly reducing the time nurses spend on administrative tasks. It can also be used to assist in document writing, e.g., grammar and spelling checks. - Personalised patient care
GenAI has the potential to provide personalised health recommendations and coaching based on patient data, most notably for chronic conditions, helping patients adhere to treatment plans more effectively. However, patient confidentiality must always be maintained, and patient personal information should not be fed into the GenAI text input. - Enhanced learning and training
The most notable use of GenAI is its application to learning and training for nurses. GenAI can recreate simulated learning scenarios to assist nursing students and help them develop their critical judgment and clinical skills. - Nursing research and innovation
Data-driven research required for continuous safe nursing practice requires time and resources. GenAI has the potential to analyse large datasets in research to identify trends, outcomes, and correlations, driving innovation in nursing practices and healthcare delivery.
Challenges and considerations
Using artificial intelligence in nursing and healthcare practice comes with several challenges and considerations that must be addressed to ensure effective and ethical use. The challenges posed by the use of GenAI include:
- Accuracy and reliability
While GenAI can process large amounts of data quickly and accurately, it sometimes lacks the ability to recognise nuances or make complex connections between pieces of information. These limitations can create biased decisions and incomplete or inaccurate answers (AIContentfy, 2024). - Bias and fairness
AI models such as ChatGPT can inherit biases present in the training data, which can result in unfair or discriminatory outcomes. In health care, AI used in algorithms can also inadvertently prioritise certain groups/variables over others, which can lead to the loss of fairness (Harvard Business Review, 2019). - Ethical and legal issues
GenAI can create content that is derivative of copyrighted works. It is not yet clear whether this is a copyright infringement or not due to a lack of regulatory policies in AI. GenAI models are also trained on personal data, which could pose privacy risks, especially if patient data has been disclosed. - Integration with existing systems
AI systems can be tricky to integrate seamlessly into an already existing operating system, especially in the healthcare setting. There is also the challenge of adoption resistance by health professionals in introducing new digital tools such as GenAI.
We must also recognise some of the considerations relating to AI use:
- Human oversight
remember that AI should only provide information to assist in human decision-making, not to replace it. Human oversight is essential to ensure AI recommendations are appropriate and safe. - Transparency and explainability
AI systems should be transparent and be able to explain how they arrive at a certain suggestion or recommendation. This will allow healthcare professionals to trust the system. - Ethical framework
As we are in the early stage of AI and GenAI applications in health care, establishing clear guidelines for AI use should be imperative to avoid issues such as bias, fairness, and data privacy. This will ensure the safe and appropriate use of AI in the healthcare system.
What’s on the horizon?
The future of AI and GenAI in nursing and health care is limitless to this point. It may potentially transform patient care as we know it by improving operational efficiency and advancing clinical research. We have seen what AI is capable of at this stage, but there are more developments on the horizon that can revolutionise the way we do things in health care. There are potential future applications of GenAI in education in nursing through integration with Virtual Reality (VR) and Augmented Reality (AR) in clinical skills by offering immersive and realistic patient care simulations (Australasian Institute of Digital Health [AIDH], 2024). Personalised learning platforms will also be available where GenAI can analyse the learner’s preference, progress, and performance and tailor educational content to their personal learning pace, style, and needs (AIDH, 2024). In a clinical context, AI is set to be fully integrated into existing systems to better assist with patient decision-making outcomes. Some transformative trends also include empowering patients to prompt better outcomes, in-practice solutions to alleviate provider burnout, embedding GenAI in medical devices, and ‘Big bad AI’ to support good behaviour (VSP Vision, 2024).
As we look to the future, the integration of AI and GenAI in nursing practice promises to revolutionise patient care, operational efficiency, clinical research, and education. The advancements that we have seen so far are just the beginning. The journey ahead is one of innovation, and should be something to look forward to!
AI is revolutionising health care, and nurses must stay ahead of the game. Prepare yourself with the Australian College of Nursing (ACN) Graduate Certificate in Digital Health This one-year, part-time online course provides essential knowledge in digital health within a professional, legal, and ethical framework. Enhance your skills, reflect on current practices, and develop leadership abilities. Equip yourself for future opportunities in education, management, and specialised nursing roles. Embrace the future of health care—enrol today and lead the digital transformation in nursing!
Author: Ross Pancho MACN
RN, MN (ClinEd), Cert IV (TAE40116), GC Digital Health
Ross has been working as a clinical nurse and a nurse educator for nearly 20 years in both international and Australian nursing practice & education sectors. Ross has been actively engaged with the education of internationally qualified nurses for national registration, diploma of nursing & undergraduate bachelor in nursing degree. Prior to education, he had extensive experience as a clinical nurse primarily looking after surgical-step down/acute cardiac care and vascular surgeries.
Ross’s recent role was working as a nurse educator and course coordinator for the Graduate Certificate in Nursing (Bridging and Re-entry) for IQRN at the Australian College of Nursing (ACN). Ross has also coordinated the ACN Graduate Certificate in Cosmetic and Dermatology Nursing as a postgraduate specialty until 2022. He is currently coordinating ACN’s Graduate Certificate in Digital Health and Graduate Certificate in Diagnostic and Interventional Radiology Nursing.
Ross has completed his Bachelor of Nursing (conversion) at Curtin University (WA), Master of Nursing specialising in Clinical Education at Charles Sturt University (CSU), Graduate Certificate in Digital Health at RMIT University (VIC) and is currently undertaking a Research Higher Degree as a PhD student at Central Queensland University (CQU).
With prior knowledge and speciality in undergraduate, postgraduate and international nursing education, Ross has become a senior advisor and trainer for these specialty areas and assists them externally during his free time. Finally, as a huge advocate for education, Ross enjoys time supporting students and promoting the importance of education in the nursing profession.
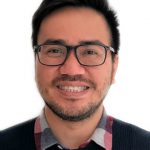
References
AIContentfy. (2024). The impact of AI on content accuracy and reliability. AIContentfy. https://aicontentfy.com/en/blog/impact-of-ai-on-content-accuracy-and-reliability
Askin, S., Burkhalter, D., Calado, G., & El Dakrouni, S. (2023). Artificial Intelligence applied to clinical trials: Opportunities and challenges. Health and Technology, 13(2), 203–213. https://doi.org/10.1007/s12553-023-00738-2
Australian Digital Health Agency (ADHA). (2016). Australia’s national digital health strategy. Australian Government.
Australasian Institute of Digital Health (AIDH). (2024). Transforming nursing and midwifery education with generative artificial intelligence. AIDH. https://digitalhealth.org.au/blog/transforming-nursing-and-midwifery-education-with-generative-ai/
Caroll, W.M. (2023). Generative AI in clinical practice and operation. Nursing Management, 54(10), 56. DOI: 10.1097/nmg.0000000000000056
eHealth NSW. (2023). Virtual care – Remote patient monitoring program. NSW Government. https://www.ehealth.nsw.gov.au/solutions/virtual-care/remote-patient-monitoring/vc-rpm
Harvard Business Review. (2019). What do we know about the biases in AI? Harvard Business Review. https://hbr.org/2019/10/what-do-we-do-about-the-biases-in-ai
HealthShare. (2013). Features and highlights eMR between the flags. NSW Government. https://www.slhd.nsw.gov.au/btf/pdfs/eMR_Features_Highlights.pdf
Hospital and Healthcare. (2023). Aust AI tool warns patient deterioration. https://www.hospitalhealth.com.au/content/technology/news/aust-ai-tool-warns-of-patient-deterioration-1531748604
Jiang, F., Jiang, Y., Zhi, H., Dong, Y., Li, H., Ma, S., Wang, Y., Dong, Q., Shen, H., & Wang, Y. (2017). Artificial intelligence in healthcare: Past, present and future. Stroke and Vascular Neurology 2(2017), 230-243. doi:10.1136/svn-2017-000101
Kaul, V., Enslin, S., & Gross, S. A. (2020). History of artificial intelligence in medicine. American Society for Gastrointestinal Endoscopy, 92(4), 807-812. Doi:10.1016/j.gie.2020.06.040.
Lundervold, A.S., & Lundervold, A. (2018). An overview of deep learning in medical imaging focusing on MRI. Z Med Phys., 29(2), 102-127. doi:10.1016/j.zemedi.2018.11.002
Rajpurkar, P., Chen, E., Banerjee, O., & Topol, E.J. (2022). AI in health and medicine. Nature Medicine, 28, 31-38. https://doi.org/10.1038/s41591-021-01614-0
Salem, H., Soria, D., Lund, J.N., & Awwad, A. (2021). A systematic review of the applications of Expert Systems (ES) and machine learning (ML) in clinical urology. BMC Medical Informatics and Decision Making, 21(223), 1-36. https://doi.org/10.1186/s12911-021-01585-9
Sloane, E. B., & J. Silva, R. (2020). Artificial intelligence in medical devices and clinical decision support systems. Clinical Engineering Handbook, 556–568. https://doi.org/10.1016/B978-0-12-813467-2.00084-5
VSP Vision. (2024). Winter 2024 Future of generative AI in healthcare. VSP Global Innovation Center. https://www.vspvision.com/dam/jcr:a1969a94-892a-4c31-8462-815255bdde43/Future%20of%20Generative%20AI%20in%20Healthcare_1%20.pdf